Saurabh Sihag
Postdoctoral Researcher at University of Pennsylvania.
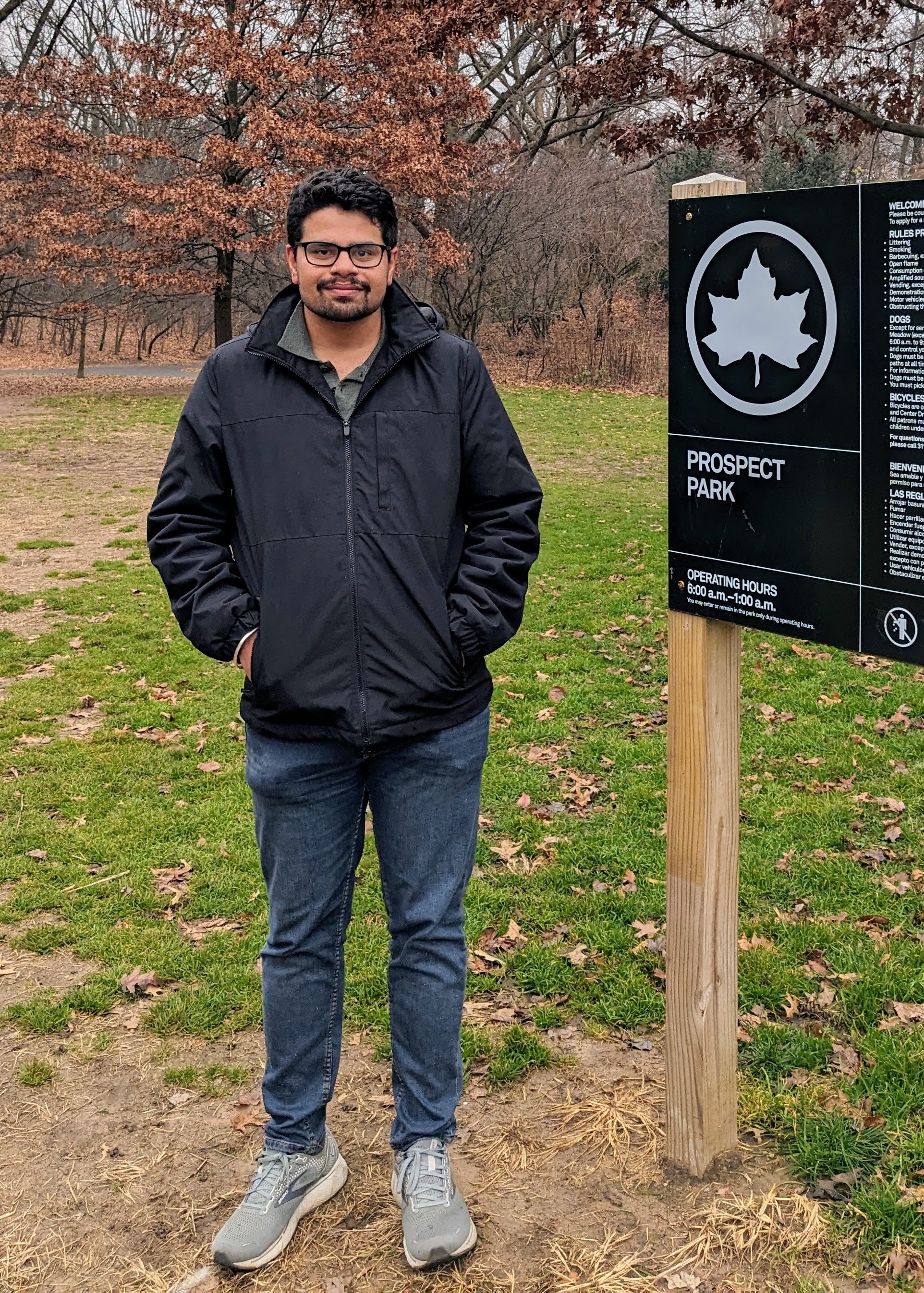
I am a postdoctoral researcher working with Dr. Alejandro Ribeiro at the University of Pennsylvania. Broadly, I study statistical inference and machine learning approaches over graph models, both from theoretic and algorithmic perspectives with applications in network neuroscience. Previously, I received the PhD degree in Electrical Engineering at Rensselaer Polytechnic Institute in Dec., 2020, where I was advised by Dr. Ali Tajer. My PhD thesis on Statistical Learning and Inference over Networks can be accessed here.
My research is interdisciplinary and spans the domains of signal processing, machine learning, neuroscience, and information theory. I have worked on a variety of research problems that include statistical learning of graph models (AISTATS, NeurIPS), state estimation in signal processing (T-IT, JSTSP, SPL), graph signal processing analyses of multimodal brain imaging data (TSIPN) and association of neuroimaging features with blood biomarkers (NatCommsMed), and adaptive graph-constrained group testing (TSP). Currently, I am investigating statistical inference using graph neural networks (VNN), with brain age prediction as a recent application (VNN_Brain_Age). A complete list of my research publications is available here.
news
March, 2024 | Paper on 'Transferability of coVariance Neural Networks' accepted at IEEE Journal of Selected Topics in Signal Processing (JSTSP) Special Series on AI in Signal & Data Science. |
---|---|
September, 2023 | Paper on 'Explainable Brain Age Prediction using coVariance Neural Networks' accepted at NeurIPS. |
June, 2023 | Paper on 'Learning Graph Structure from Convolutional Mixtures' accepted at Transactions on Machine Learning Research. |
June, 2023 | Paper on 'Predicting Brain Age using Transferable coVariance Neural Networks' was presented at ICASSP, 2023. |
Sep. 14, 2022 | Paper on 'coVariance Neural Networks' was accepted at NeurIPS, 2022. |
Sep. 1, 2022 | Paper on 'Estimating structurally similar graphical models' was accepted at IEEE Transactions on Information Theory. |
April, 2022 | Our paper on 'Summary Markov Models for Event Sequences' accepted for long presentation at International Joint Conference on Artificial Intelligence, 2022. |
April, 2022 | Abstract 'Structural Modularity is a Feature of C9orf72 Intrinsic Network Degeneration' accepted for oral presentation at Alzheimer's Association International Conference, 2022. |
Dec 10, 2021 | Our paper on Adaptive Graph-constrained Group Testing was accepted at IEEE Transactions on Signal Processing. |
Dec 7, 2021 | Our paper on Functional Brain Signals Constrained by Structural Brain Connectivity Reveal Cohort Specific Features for Serum Neurofilament Light Chain was accepted at Communications Medicine. |
Nov 9, 2021 | Abstract on De-differentiation of functional networks in corticobasal syndrome was presented virtually at Society for Neuroscience, 2021. |